Dynamic Customer Segmentation: The Next Evolution in Marketing
The current state of customer segmentation is quite antiquated in an age where customers expect immediate relevant communication from brands regularly. The Accenture research statistics outlined below reveal the “state of irrelevancy” of many brands today.
Not being relevant with customers is very costly is several ways – only switching costs stand in the way of inevitably losing customers to those that are more relevant. For example, the finding that 64% of the instances when customers switch from one brand to another is driven by a lack of relevance and that 1 in 4 customers say they’d quit doing business altogether with a company that isn’t relevant.
Couple that with the fact that high value customers drive an extremely high percentage of a company’s revenue and profits – you can’t escape the need to “get relevant” as quickly as possible. One way to do this is to upgrade your customer segmentation with AI.
We explored this topic in depth in a sponsored research report, Capture the Customer Moment with Dynamic Predictive Segmentation, January 2018. Although 100% of firms interviewed agree its risky not to adopt dynamic customer segmentation, very few have it.
Upgrading to Dynamic Segmentation
All businesses use some form of segmentation to communicate with their customers. Your customers aren’t static, and your segmentation models shouldn’t be either! However, most brands still use a fixed group of descriptive, static segments that are updated on a very slow schedule.
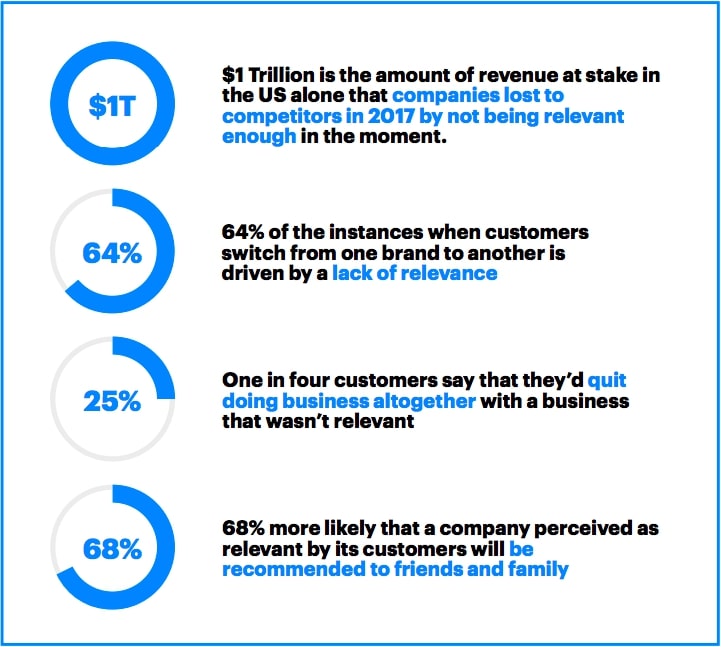
Achieving Sustainable Growth Through Hyper Relevance, Accenture, 8/18
The problem with using 5-8 static segments today is that they don’t keep up with customer needs. This is the first and biggest challenge businesses face in solving their relevancy problem. Companies need to move to dynamic customer segmentation to achieve a much higher degree of relevancy in the moment of every customer interaction.
What is Dynamic Segmentation?
Having a common definition of what dynamic customer segmentation, or Dynamic Predictive Segmentation (DPS) as it’s referred to in the report, would be a good starting point.
The Forrester report describes DPS as follows:
DPS is an approach that segments customers based on propensities to take a specific action grouped by the most important shared characteristics revealed for each segment. Segments and their associated characteristics change dynamically in real-time, based on new data from customer interactions. Therefore, this type of segmentation is dynamic in multiple ways because not only do customers’ segment assignments change as their behaviors change, but their propensities also update in real time as well. Advances in “explainable AI” have made the factors leading to these segments transparent, enabling a new level of contextual relevancy in customer interactions.
If we break this definition down to its core, DPS is predictive vs. descriptive and dynamic vs. static.
It updates based on new data based on which machine learning-driven segment a customer fits into and reveals the factors associated with predicted behavior in order of importance. This provides insight into what is relevant to that customer segment.
Who wouldn’t want to deploy that? Apparently, no one wouldn’t; 100% agree it’s risky not to do adopt a dynamic segmentation model according to the report. So what’s the problem?
There are many obstacles we have found but the main one is organizational inertia or lack of a pathway to move from a very rigid and brittle current state to this new type of Dynamic Predictive Segmentation.
How to Improve Your Customer Segmentation Models
There is a logical roadmap to migrate from current to future state in the world of customer segmentation. Adopting an explainable machine learning customer segmentation model is part of that roadmap from beginning to end. AI offers powerful alternatives to traditional statistical techniques, so as companies become aware of this technology, I am going to provide a simple high level two step process to this evolution.
Step One: Use XAI to Create the Same Number of Segments In Place Now
One of the first ways to get started down the path of using better explainable AI (XAI) driven segmentation is to focus on keeping fewer segments in place –to smooth out the operational impact of using a different analytic software to create segments. By doing this you automatically can become more relevant with customers as outlined in the table below:
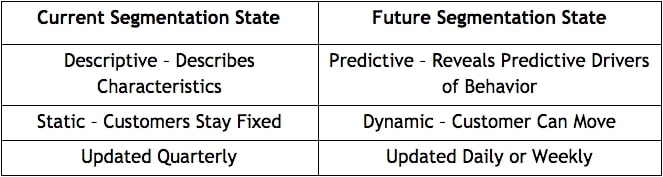
Track these movements by simply capturing segment assignment history by customer. As you rotate different offers to them, you can think about how that affects messaging over time. But simply updating more frequently will assign customers to the most relevant segment at the moment given updated data.
Training an XAI algorithm on the same underlying data as your original clusters were created from with a target number of K segments will produce the same number of segments – except they will be machine driven and predictive – showing the predictive factors associated with each segment. There are a few immediate things you should do once you have replicated the same number of segments with XAI:
1. Examine how the Segments are Different
The segments may be similar in that the XAI technology is still grouping through K-means, similar objects. However the technology can divide clusters by predicted class – “will buy” or “won’t buy” for example. What are the customer counts by segment?
2. What Are the Predictive Drivers of Each Segment
These new segments will show predictive drivers of behavior. This is where a great deal of insight can be gleaned with regard to what is important to those customers predicted to buy or not buy. How does this affect your communication to them?
3. Make Simple Tweaks to Offers and Messages to Start
Using the above insights, you can make simple updates to existing copy if necessary, where predictive factors are compelling and clear. These can be very subtle but meaningful in simply acknowledging in some what is most important to a group of customers.
4. Ask Yourself What Data Is Missing
Based on these predictive factors, ask yourself if you have fed into the analytic software all the data you should have available.
For example, primary research data can be modeled out across customers and then included in segmentation as factors that could in fact be predictive. Or you can report all the descriptive characteristics out associated with new predictive segments, and examine the intersection of predictive and descriptive characteristics to think through how your customers are really behaving and what defines them.
5. Update More Frequently
Since XAI can update predictive clusters at a faster rate than traditional segmentation models, you can see how customers move from one segment to another across updates.
Step Two: Expand the Number of Segments by Increasing K Slowly Over Time
Expanding K is easy with XAI. You can re-run segmentation and just change the settings to set K at whatever you want it to be. However, what do you do with new segments?
Obviously, absorbing new segments into your existing campaign process is not a trivial task. New creative and media planning needs to take place based on these new segments. So just sticking with the customer side of the equation for now there are specific steps you should consider in expanding K. These are as follows:
1. Examine New Segments Compared to Existing Segments
These new segments will now reflect existing customers that have moved from the old segments into these new machine-driven segments. This is simply a tighter clustering of objects that cluster together more discreetly than before.
The new predictive features and descriptive factors that define them will be distinct. A comparison of the factors between new segments vs. old can be done to assess what makes these segments different.
2. Generate New Treatments for New Segments One at a Time
You can develop new treatments and media strategies on your timeline while executing against the original segments. Work with your agency to inform new creative briefs for a handful of new segments that seem discrete and meaningful in size – warranting the investment.
3. Test and Learn Against New Segments
These new segments can be activated one at a time as well into the campaign stream through testing. Running A/B tests against these new segments will help examine performance over time.
Since these are predictive segments, targeting predicted buyers for example with relevant offers to their weighted factors should outperform standard response and conversion metrics. A/B testing will help refine both messaging and offers based on different treatments over time to optimize yield.
4. Evaluate What is the Optimal K in Non-Production Over Time
Running multiple segmentation scenarios in a development server off to the side for analysis and planning is always a good idea. As customers evolve, data changes and you get more sophisticated at matching treatments to more segments and understanding where best to reach these customers in terms of channels, you will be well informed about how to evolve your segmentation by increasing K further. Ultimately you will level off to the right number for optimal gain.
5. Consider Multiple Segmentation Strategies for Different Parts of the Life Cycle
Since you can, you may want to run segmentations related to increased product usage, cross sell, upsell, churn and reactivation independently of one another. As a customer moves across the lifecycle horizon – you can be even more relevant through this approach. However, this assumes your organization has kept up with being customer aligned in a way that you can actually operationalize such a sophisticated approach.
Dynamic Segmentation is Easier Than You Think
The Important Thing is to get started sooner than later. Leading firms are starting to implement this type of approach. It’s not as daunting as a project as it may first appear. Why? Because XAI technology does a lot of the heavy lifting so you can spend your time analyzing what it’s telling you.
This technology does not require scores of analysts – just a location to place the software and data together in the same place and letting the machine run various outputs. Innovative marketers are starting to test and evaluate this technology to ensure that they can start to keep pace with their customers before they are stolen away by others that have already started.