Scientific Breakthroughs in Similarity Machine Learning
Similarity based machine learning is a preferred method for marketing, fraud, compliance and a range of other business applications because of its ability to provide explainable AI outputs. However, this method has been unable to scale to large volumes of data, until now. simMachines achieved several technology innovations enabling our proprietary similarity based machine learning methods to operate at unlimited speed and scale.
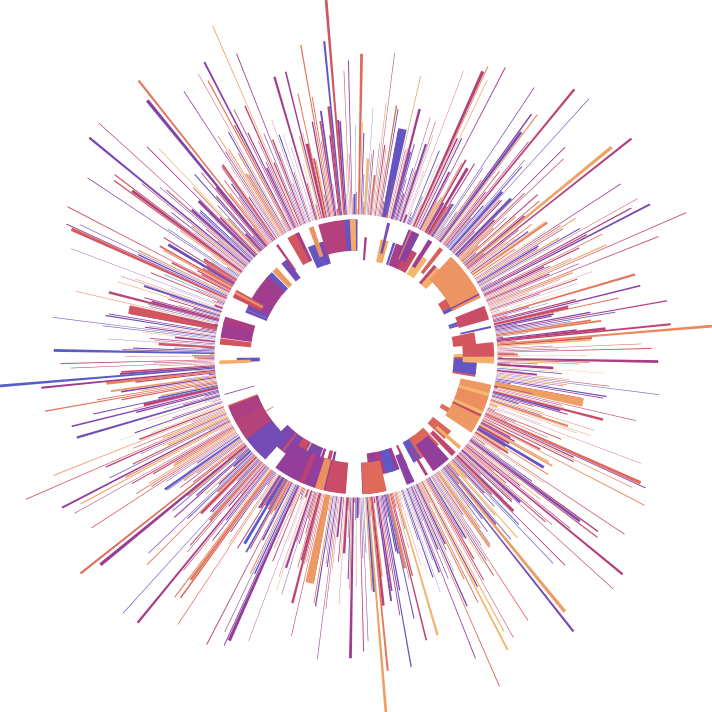
Proprietary Technology Innovations
Several technological breakthroughs enable simMachines vast performance gains over traditional nearest neighbor approaches. As a result, simMachines can match or outperform any other machine learning method in both prediction accuracy and precision, while providing “the why” behind every prediction.
Data scientists can assess simMachines performance through a technology evaluation that will demonstrate performance over current state and ground truth.
These advancements include:
METRIC OR NON-METRIC DISTANCE FUNCTIONS
Provides significant improvements in accuracy over traditional Euclidean distance
DYNAMIC FACTOR REDUCTION
Enables a response time in the millisecond range on a dataset of any size or shape
verifyAI: simMachines Engines at Work
simMachines provides Data Scientists with access to multiple modeling engines through the verifyAI software interface. Each engine performs different tasks and are sometimes used in unison, based upon the project objective.

simSearch
Drives nearest neighbor distance calculations in “n” dimensional space. simSearch is foundational for retaining the Why behind a predicted outcome. A library of distance functions provide flexible options to data scientists.

simClassify
simClassify creates predictions by assigning a predicted outcome to a class. Simple or complex predictions can be supported with full justification at the local level. simClassify can reveal the Why factors behind other algorithms by mirroring their predictions. Multiple predictions can be used together to solve specific problems.

simCluster
simCluster uses unsupervised clustering for analysis and data exploration and supervised clustering to create dynamic predictive segments. Parameters and distance functions can be adjusted by the user to refine segments. Dynamic predictive segments groups objects based by the most important shared characteristics for each segment which are revealed in weighted factor order. Segments and their associated characteristics change dynamically and in real-time based on new data.

simRecommend
simRecommend drives dynamic recommendations in real time for optimizing customer interactions. simRecommend provides the Why factors to enable context and relevancy to be dynamically adjusted and applied during each interaction.
Research, Benchmarks & White Papers
simMachines has participated in and written a number of white papers and performance benchmark summaries contained here, specifically for data scientists. Additionally, third party research on the subject of machine learning performance is also included.