Fighting Fraud with Dynamic Predictive Segmentation
The rapidly changing landscape of fraud requires a tool that can swiftly adapt to emergent threats. simMachines’ Dynamic Predictive Segmentation provides a highly accurate way to identify threats new and old by coupling cutting edge accuracy with unparalleled transparency and continuous learning. The union of these powerful features results in a tool that not only provides top-tier fraud detection but also enables customers to proactively combat fraud by identifying the underlying factors that are evidence of fraud for each transaction. For this demonstration we’ve trained a classifier with a credit card transaction dataset labeled with transaction state: fraud / normal and built clusters around that classifier. The result is highly accurate predictive clusters that identify the different types of fraud being committed. Let’s take a dive into the clusters.
Data Overview
Let’s take a look at the largest fraud cluster:
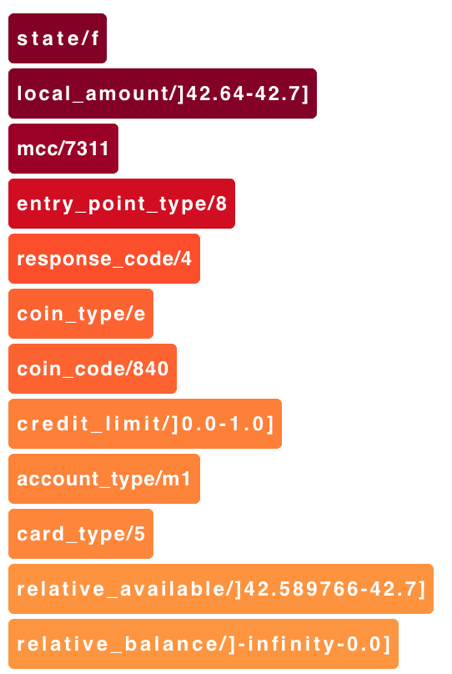

We see that there are a large number (based on the count and width of the cluster) of lower value (~$42) transactions are being processed through vendors using MCC (merchant category code) 7311 – an indicator identifying the card as being processed by a merchant offering advertising services. Our system has learned that legitimate transactions with MCC 7311 are typically of higher value and are normally processed through an Entry Point Type of 9 or 1.
Now let’s look at a different fraud cluster:


For this cluster we see that the factors indicating these transactions as fraudulent are significantly different! Transactions with MCC 7994 (transactions at a video arcade) we’ve identified a number of very high value transactions with Response Code 58 and Entry Point Type 8.