Machine Learning for Financial Services
Financial Services companies need to continuously advance fraud detection capabilities and provide transparency behind every credit and loan decision made to comply with current or pending legislation. simMachines provides game changing machine learning software that drives significant marketing performance lift, fraud detection and compliance with full transparency behind every prediction.

“We have a whole department that gives a propensity to take an action on each of our accounts. If there’s something that can give us a better answer, it would be incredibly valuable.”
– Sr. Manager of Marketing Strategy, Financial company
Capture The Customer Moment With Dynamic Predictive Segmentation, a January 2018 commissioned study conducted by Forrester Consulting on behalf of simMachines
Revealing the Why Behind Every Prediction
simMachines provides the following applications for global financial institutions, banks and insurance companies. We provide the Why factors behind every prediction and can expose the Why behind any other machine learning method. simMachines is the only machine learning technology that can provide transparency behind every prediction at the local vs. global level.

Dynamic Predictive Card/Load Holder Segmentation

Customer Opinion Clustering
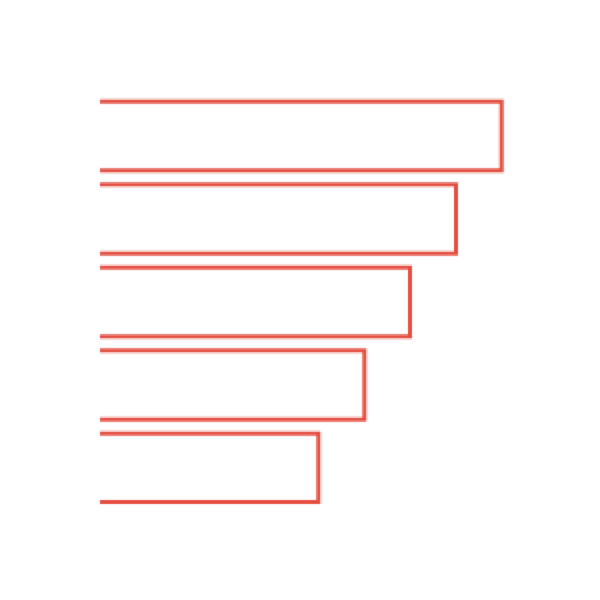
Customer Lifecycle Predictions

Fraud Prevention

Product Demand Predictions

Customer Experience Predictions

Risk Analysis

Legislative Compliance
See it in action
Use Cases
simMachines supports financial services clients across marketing, fraud and compliance use cases. Fraud detection machine learning is a primary area that financial service companies benefit from knowing why fraud is occurring, to adjust business strategies for future prevention.

Customer Acquisition Credit Worthiness
An international bank client provides loans to small businesses. Our client needed a custom, predictive engine that would help quickly determine the credit worthiness of a small business owner.

Machine Learning Fraud Detection
Top 3 financial institution wanted to speed up ability to implement ML fraud detection solutions for clients, enable continuous learning, and expose the factors driving the fraud.

Compliance Monitoring
Global Exchange company deployed method of monitoring OTC submissions for rules-infringing transactions lacked sensitivity and required significant human capital.
Recent news in financeALL NEWS ON OUR BLOG
Dynamic Customer Segmentation: The Next Evolution in Marketing The current state of customer segmentation is quite antiquated in an age where customers expect immediate relevant communication from brands regularly.
Advertising is more data driven today than ever before. Digital advertising has evolved over the last ten years to define audiences at much greater levels of granularity.
Advertising is more data driven today than ever before. Digital advertising has evolved over the last ten years to define audiences at much greater levels of granularity.